Experimental Particle Physics
Experimental particle physics is the practical way to look for the fundamental components of matter (like quarks). An important tool for the experimental particle physicist is the particle accelerator which speeds up the fundamental particles close to the light speed. Particles then collide together to reveal the mystery inside the matters. Other crucial tools in this field are detector/interaction simulators, data driven techniques, and statistical packages. The world’s major particle physics laboratories are: CERN, DESY, Fermilab, and SLAC.
Theoretical Particle Physics
Theoretical particle physics is the development of models for describing fundamental particles and their interactions. This includes testing and validating the Standard Model (SM) and the beyond Standard Models (like Supersymmetry). Particles are described as excitations of quantum fields in the Standard Model. Crucial tools in the arsenal of the theoretical particle physicist are group theory, quantum field theory, and effective field theory. In general, theorists attempt to construct a unified quantum mechanics and general relativity.
LHC accelerator and ATLAS detector
Using particle accelerators to produce and detect new particles
The Large Hadron Collider (LHC) is the world’s largest and highest-energy particle collider which was built by the European Organization for Nuclear Research (CERN). It is a circular hadron (like proton) accelerator, located in a 26.7 km long underground tunnel. ATLAS is the largest of the LHC detectors, measuring 44 meters in high, and 25 meters in height. The ATLAS physics program covers variety of topics such as discovery of Higgs boson, measuring the SM parameters, and studying of beyond the Standard Model physics. This collaboration includes over 10,000 scientists and hundreds of universities and laboratories, as well as more than 100 countries.
How it works:
Machine Learning model modifies its rules based on the errors it measures
Data Preprocessing
Summary of root to CSV file conversion
Model Training and Overfitting Check
Neural network and Random forest models
Model Comparison
Evaluating models and picking the model with the highest precision and recall
Machine Learning application in detecting Beyond the Standard Model signals
HEP Projects
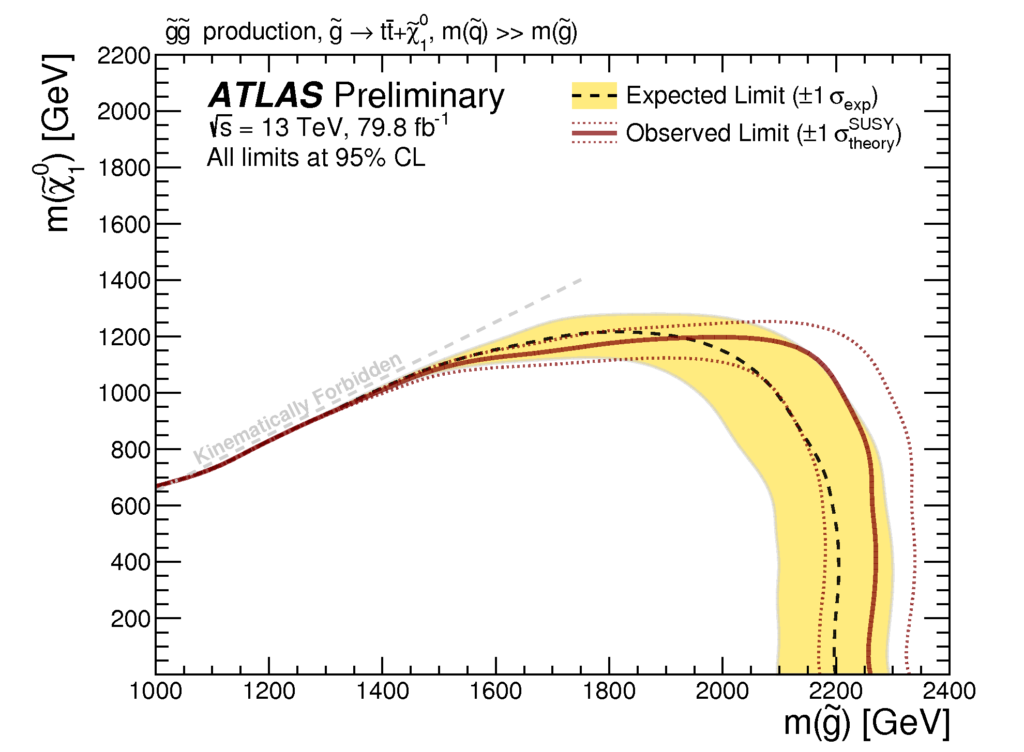
SUSY Strong multi-B
The search is performed for gluino pair production, leading to the final states with multiple top and/or bottom quarks and ETmiss. 139 fb-1 integrated luminosity in this analysis is collected with the ATLAS detector from 2015 to 2018. Gluino masses of less than 2.3 TeV are excluded at 95% confidence level.
Key words: Gluino, Neutralino, Cut-and -count, Neural network, Exclusion fit.

SUSY Electroweak multi-B
The search is performed for Higgsino pair production, with decay to Higgs or Z boson, focusing on the final states with four bottom quarks and ETmiss. Data collected from 2015 to 2018 is used and Higgsino masses of less than 2.1 TeV are excluded at 95% confidence level.
Key words: Higgsino, Neutralino, Low and High mass regions, Neural network, BDT, Exclusion fit.
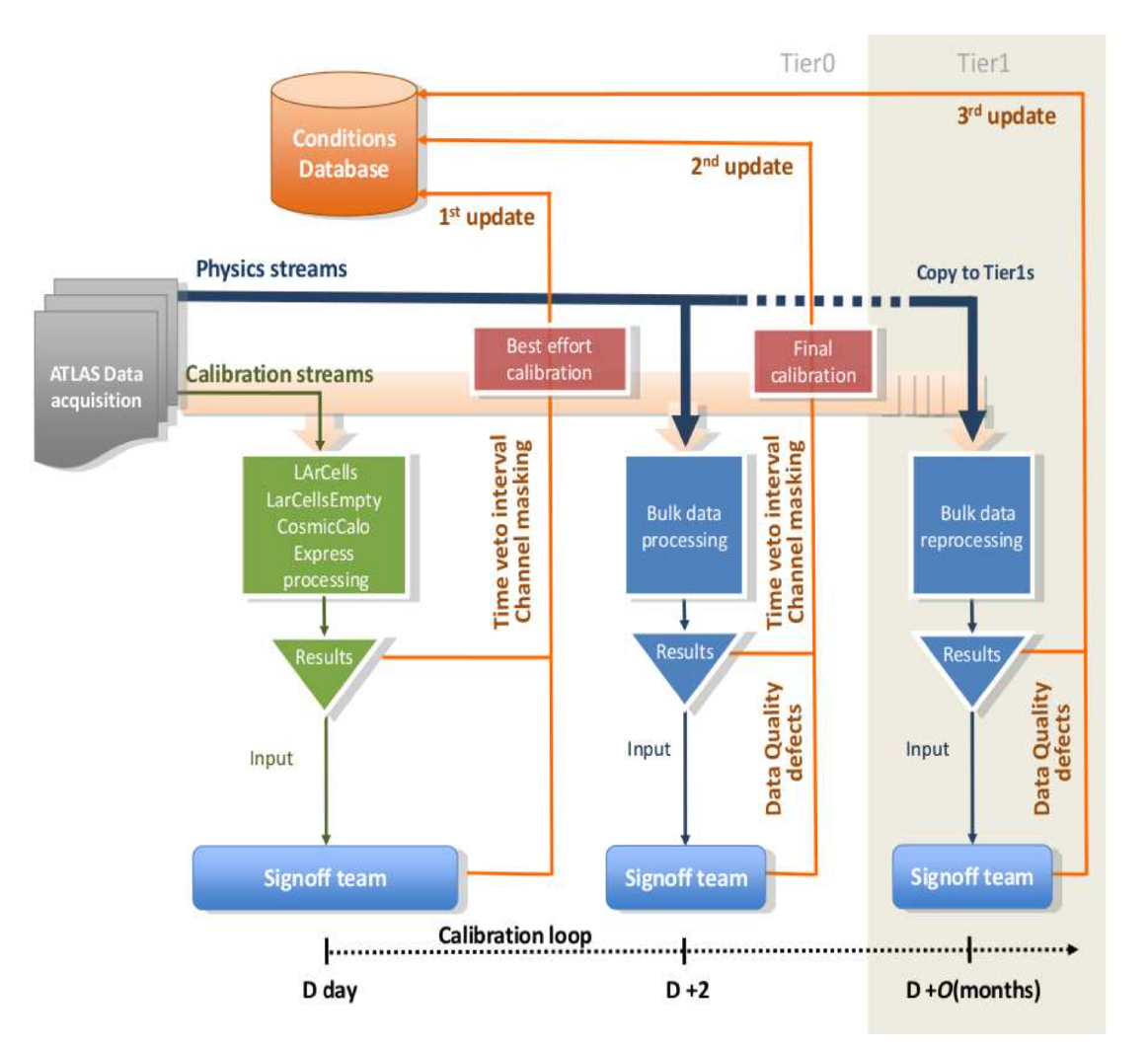
ATLAS LAr Data Quality
To ensure the highest quality LAr calorimeter data with minimal data rejection during physics analyses, an advanced data quality monitoring system has been developed. This system employs a sophisticated flagging scheme, applied at both offline and online levels, to accurately identify and manage noisy channels.
Key words: Noisy channels, UPD soft, LTTNK package.

Online Calo desk shift
The individual on shift holds the primary responsibility for assessing the quality of data acquisition and is best equipped to address any anomalies if the data is suboptimal. This critical duty underscores the level of responsibility entrusted to shifters in the ATLAS control room. Their vigilance directly impacts the integrity of the data used for physics analyses.
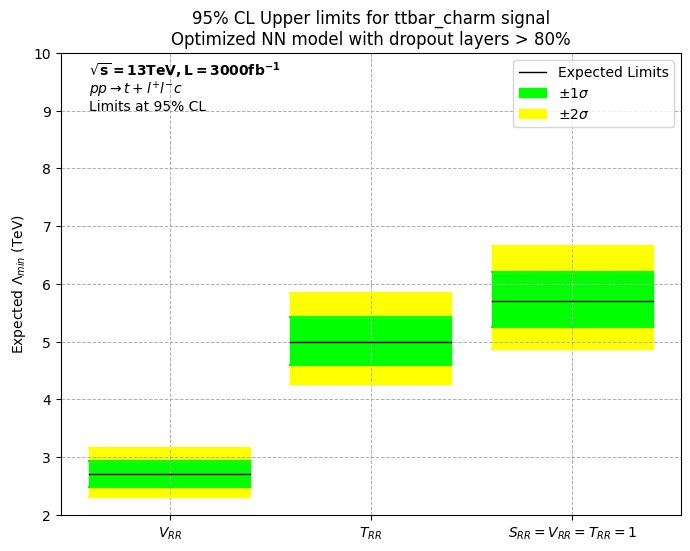
Top Flavor changing using ML
the top quark flavor changing neutral current (FCNC) interaction is investigated using machine learning models through the tri-electron processes at the LHC for center-of-mass energy of 14 TeV. The expected 95% CL bound on the scale of new physics is Λ > 5.7 TeV for tcee process.
Key words: Flavor changing, Deep Neural network, Random forest, Hyperparameter, Upper limit. Github link.
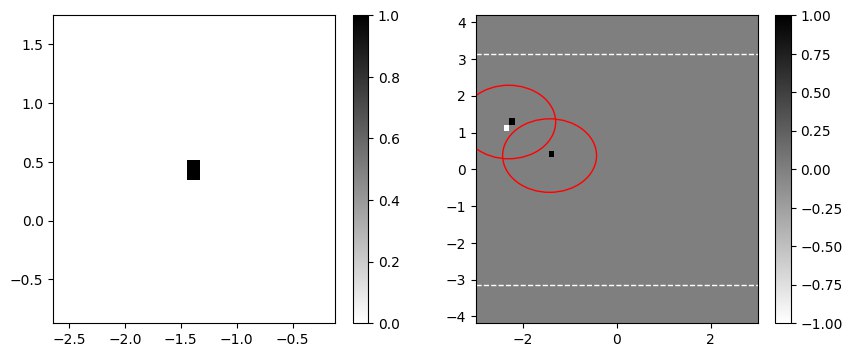
QML for Jet Charge discrimination
Focused on enhancing jet charge discrimination between up and anti-up quarks by utilizing deep neural networks and convolutional networks. Additionally, I explored the application of quantum machine learning models, including Quantum Support Vector Machines (QSVM) and Quantum Neural Networks (QNN), to further improve classification accuracy and investigate the potential advantages of quantum computing in particle physics analyses.
Key words: Deep Neural network, Convolutional model, Quantum machine learning, Quantum support vector machine. Github link.
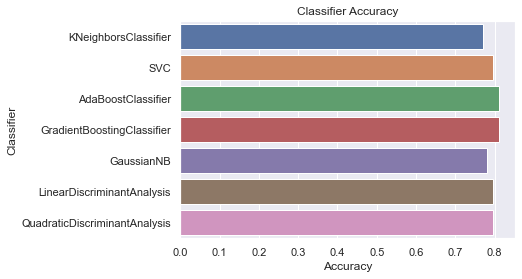
Unraveling Academic Success Using Machine Learning
In two studies, I applied Machine Learning to predict GPA and analyze demographic diversity among Tehran University students. The first study used algorithms like Random Forests and neural networks, with the optimized Random Forest achieving the lowest RMSE of 1.53, improving accuracy by 3%. The second study differentiated tuition-paying from tuition-free students, with the optimized neural network achieving an 82% precision rate and an F1 score of 0.89. Github link.

Anomaly Detection
Anomaly detection in particle physics using Variational Autoencoders (VAEs) represents a promising unsupervised learning approach for identifying rare or unexpected events that deviate from the Standard Model predictions. VAEs are generative models that learn a compressed latent representation of high-dimensional input data, such as detector-level features or jet images, by optimizing a reconstruction loss combined with a regularization term that enforces a smooth latent space. Github link.